How to show for any orthogonal vector set and any matrix $sum_u |Au|_2^2 leq |A|_F^2$?
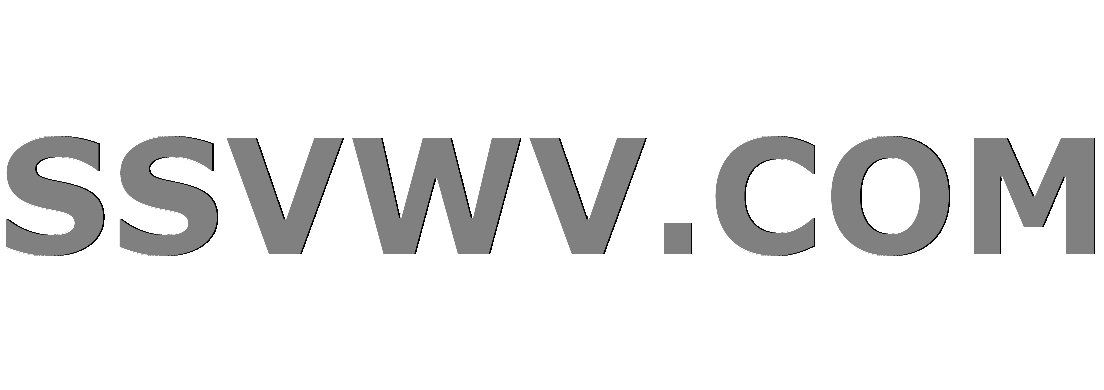
Multi tool use
The following paper on page 16, in line 17
Online Principal Component Analysis
says for any orthogonal vector set and any matrix $sum_u |Au|_2^2 leq |A|_F^2$ is true. However, if we let $u_i$'s in $mathbb{R}^m$ be a set of orthogonal vectors and $A in mathbb{R}^{n times m}$ we have
$$sum_u |Au|_2^2=|AU|_F^2$$
where $U$ is the matrix that has $u_i$ as its columns and $|cdot|_F$ is Frobenius norm. Using Cauchy-Schwarz inequality
$$|AU|_F^2 leq |A|_F^2|U|_F^2$$
My question is how we can ignore $|U|_F^2$?
linear-algebra cauchy-schwarz-inequality
add a comment |
The following paper on page 16, in line 17
Online Principal Component Analysis
says for any orthogonal vector set and any matrix $sum_u |Au|_2^2 leq |A|_F^2$ is true. However, if we let $u_i$'s in $mathbb{R}^m$ be a set of orthogonal vectors and $A in mathbb{R}^{n times m}$ we have
$$sum_u |Au|_2^2=|AU|_F^2$$
where $U$ is the matrix that has $u_i$ as its columns and $|cdot|_F$ is Frobenius norm. Using Cauchy-Schwarz inequality
$$|AU|_F^2 leq |A|_F^2|U|_F^2$$
My question is how we can ignore $|U|_F^2$?
linear-algebra cauchy-schwarz-inequality
1
$|AU|_F=|A|_F$ which follows from the trace property and $UU^*=I$.
– A.Γ.
2 days ago
1
But they are not orthanormal, it says they are orthogonal.
– Saeed
2 days ago
Some authors are sloppy about the distinction between "orthonormal" and "orthogonal". It's pretty straight-forward to normalize orthogonal vectors (orthogonalizing them using, say, Gram-Schmidt, is what takes all the effort!), so some authors don't trouble about it. Technically, you're correct, of course.
– Adrian Keister
2 days ago
2
It is sloppy written, but $U$ matrix they work with in this proof has orthonormal columns (Observation 14, page 14). Otherwise it is not true (take one vector and multiply it by a huge number).
– A.Γ.
2 days ago
add a comment |
The following paper on page 16, in line 17
Online Principal Component Analysis
says for any orthogonal vector set and any matrix $sum_u |Au|_2^2 leq |A|_F^2$ is true. However, if we let $u_i$'s in $mathbb{R}^m$ be a set of orthogonal vectors and $A in mathbb{R}^{n times m}$ we have
$$sum_u |Au|_2^2=|AU|_F^2$$
where $U$ is the matrix that has $u_i$ as its columns and $|cdot|_F$ is Frobenius norm. Using Cauchy-Schwarz inequality
$$|AU|_F^2 leq |A|_F^2|U|_F^2$$
My question is how we can ignore $|U|_F^2$?
linear-algebra cauchy-schwarz-inequality
The following paper on page 16, in line 17
Online Principal Component Analysis
says for any orthogonal vector set and any matrix $sum_u |Au|_2^2 leq |A|_F^2$ is true. However, if we let $u_i$'s in $mathbb{R}^m$ be a set of orthogonal vectors and $A in mathbb{R}^{n times m}$ we have
$$sum_u |Au|_2^2=|AU|_F^2$$
where $U$ is the matrix that has $u_i$ as its columns and $|cdot|_F$ is Frobenius norm. Using Cauchy-Schwarz inequality
$$|AU|_F^2 leq |A|_F^2|U|_F^2$$
My question is how we can ignore $|U|_F^2$?
linear-algebra cauchy-schwarz-inequality
linear-algebra cauchy-schwarz-inequality
asked 2 days ago
Saeed
698310
698310
1
$|AU|_F=|A|_F$ which follows from the trace property and $UU^*=I$.
– A.Γ.
2 days ago
1
But they are not orthanormal, it says they are orthogonal.
– Saeed
2 days ago
Some authors are sloppy about the distinction between "orthonormal" and "orthogonal". It's pretty straight-forward to normalize orthogonal vectors (orthogonalizing them using, say, Gram-Schmidt, is what takes all the effort!), so some authors don't trouble about it. Technically, you're correct, of course.
– Adrian Keister
2 days ago
2
It is sloppy written, but $U$ matrix they work with in this proof has orthonormal columns (Observation 14, page 14). Otherwise it is not true (take one vector and multiply it by a huge number).
– A.Γ.
2 days ago
add a comment |
1
$|AU|_F=|A|_F$ which follows from the trace property and $UU^*=I$.
– A.Γ.
2 days ago
1
But they are not orthanormal, it says they are orthogonal.
– Saeed
2 days ago
Some authors are sloppy about the distinction between "orthonormal" and "orthogonal". It's pretty straight-forward to normalize orthogonal vectors (orthogonalizing them using, say, Gram-Schmidt, is what takes all the effort!), so some authors don't trouble about it. Technically, you're correct, of course.
– Adrian Keister
2 days ago
2
It is sloppy written, but $U$ matrix they work with in this proof has orthonormal columns (Observation 14, page 14). Otherwise it is not true (take one vector and multiply it by a huge number).
– A.Γ.
2 days ago
1
1
$|AU|_F=|A|_F$ which follows from the trace property and $UU^*=I$.
– A.Γ.
2 days ago
$|AU|_F=|A|_F$ which follows from the trace property and $UU^*=I$.
– A.Γ.
2 days ago
1
1
But they are not orthanormal, it says they are orthogonal.
– Saeed
2 days ago
But they are not orthanormal, it says they are orthogonal.
– Saeed
2 days ago
Some authors are sloppy about the distinction between "orthonormal" and "orthogonal". It's pretty straight-forward to normalize orthogonal vectors (orthogonalizing them using, say, Gram-Schmidt, is what takes all the effort!), so some authors don't trouble about it. Technically, you're correct, of course.
– Adrian Keister
2 days ago
Some authors are sloppy about the distinction between "orthonormal" and "orthogonal". It's pretty straight-forward to normalize orthogonal vectors (orthogonalizing them using, say, Gram-Schmidt, is what takes all the effort!), so some authors don't trouble about it. Technically, you're correct, of course.
– Adrian Keister
2 days ago
2
2
It is sloppy written, but $U$ matrix they work with in this proof has orthonormal columns (Observation 14, page 14). Otherwise it is not true (take one vector and multiply it by a huge number).
– A.Γ.
2 days ago
It is sloppy written, but $U$ matrix they work with in this proof has orthonormal columns (Observation 14, page 14). Otherwise it is not true (take one vector and multiply it by a huge number).
– A.Γ.
2 days ago
add a comment |
1 Answer
1
active
oldest
votes
Note that $A^*A$ is a square matrix and $A^*A ge 0$ so there exists an orthonormal basis ${u_1, ldots, u_m}$ for $mathbb{R}^m$ such that $A^*A u_i = lambda_i u_i$ for some $lambda ge 0$.
We have
$$sum_{i=1}^m |Au_i|_2^2 = sum_{i=1}^m langle Au_i, Au_irangle = sum_{i=1}^m langle A^*Au_i, u_irangle = sum_{i=1}^m lambda_i =operatorname{Tr}(A^*A) = |A|_F^2$$
The interesting part is that the sum $sum_{i=1}^m |Au_i|_2^2$ is actually independent of the choice of the orthonormal basis ${u_1, ldots, u_m}$. Indeed, if ${v_1, ldots, v_m}$ is some other orthonormal basis for $mathbb{R}^m$, we have
begin{align}
sum_{i=1}^m |Au_i|_2^2 &= sum_{i=1}^m langle A^*Au_i, u_irangle\
&= sum_{i=1}^m leftlangle sum_{j=1}^mlangle u_i,v_jrangle A^*A v_j , sum_{k=1}^mlangle u_i,v_krangle v_krightrangle\
&= sum_{j=1}^m sum_{k=1}^m left(sum_{i=1}^mlangle u_i,v_jrangle langle v_k,u_irangleright)langle A^*A v_j,v_krangle\
&= sum_{j=1}^m sum_{k=1}^m langle v_j,v_kranglelangle A^*A v_j,v_krangle\
&= sum_{j=1}^m langle A^*A v_j,v_jrangle\
&= sum_{j=1}^m |Av_j|_2^2
end{align}
Hence we can extend any orthonormal set ${v_1, ldots, v_k} subseteq mathbb{R}^m$ to an orthonormal basis ${v_1, ldots, v_m}$ for $mathbb{R}^m$ to obtain
$$sum_{i=1}^k |Av_i|_2^2 lesum_{i=1}^m |Av_i|_2^2 = sum_{i=1}^m |Au_i|_2^2 = |A|_F^2 $$
add a comment |
Your Answer
StackExchange.ifUsing("editor", function () {
return StackExchange.using("mathjaxEditing", function () {
StackExchange.MarkdownEditor.creationCallbacks.add(function (editor, postfix) {
StackExchange.mathjaxEditing.prepareWmdForMathJax(editor, postfix, [["$", "$"], ["\\(","\\)"]]);
});
});
}, "mathjax-editing");
StackExchange.ready(function() {
var channelOptions = {
tags: "".split(" "),
id: "69"
};
initTagRenderer("".split(" "), "".split(" "), channelOptions);
StackExchange.using("externalEditor", function() {
// Have to fire editor after snippets, if snippets enabled
if (StackExchange.settings.snippets.snippetsEnabled) {
StackExchange.using("snippets", function() {
createEditor();
});
}
else {
createEditor();
}
});
function createEditor() {
StackExchange.prepareEditor({
heartbeatType: 'answer',
autoActivateHeartbeat: false,
convertImagesToLinks: true,
noModals: true,
showLowRepImageUploadWarning: true,
reputationToPostImages: 10,
bindNavPrevention: true,
postfix: "",
imageUploader: {
brandingHtml: "Powered by u003ca class="icon-imgur-white" href="https://imgur.com/"u003eu003c/au003e",
contentPolicyHtml: "User contributions licensed under u003ca href="https://creativecommons.org/licenses/by-sa/3.0/"u003ecc by-sa 3.0 with attribution requiredu003c/au003e u003ca href="https://stackoverflow.com/legal/content-policy"u003e(content policy)u003c/au003e",
allowUrls: true
},
noCode: true, onDemand: true,
discardSelector: ".discard-answer"
,immediatelyShowMarkdownHelp:true
});
}
});
Sign up or log in
StackExchange.ready(function () {
StackExchange.helpers.onClickDraftSave('#login-link');
});
Sign up using Google
Sign up using Facebook
Sign up using Email and Password
Post as a guest
Required, but never shown
StackExchange.ready(
function () {
StackExchange.openid.initPostLogin('.new-post-login', 'https%3a%2f%2fmath.stackexchange.com%2fquestions%2f3060689%2fhow-to-show-for-any-orthogonal-vector-set-and-any-matrix-sum-u-au-22-leq%23new-answer', 'question_page');
}
);
Post as a guest
Required, but never shown
1 Answer
1
active
oldest
votes
1 Answer
1
active
oldest
votes
active
oldest
votes
active
oldest
votes
Note that $A^*A$ is a square matrix and $A^*A ge 0$ so there exists an orthonormal basis ${u_1, ldots, u_m}$ for $mathbb{R}^m$ such that $A^*A u_i = lambda_i u_i$ for some $lambda ge 0$.
We have
$$sum_{i=1}^m |Au_i|_2^2 = sum_{i=1}^m langle Au_i, Au_irangle = sum_{i=1}^m langle A^*Au_i, u_irangle = sum_{i=1}^m lambda_i =operatorname{Tr}(A^*A) = |A|_F^2$$
The interesting part is that the sum $sum_{i=1}^m |Au_i|_2^2$ is actually independent of the choice of the orthonormal basis ${u_1, ldots, u_m}$. Indeed, if ${v_1, ldots, v_m}$ is some other orthonormal basis for $mathbb{R}^m$, we have
begin{align}
sum_{i=1}^m |Au_i|_2^2 &= sum_{i=1}^m langle A^*Au_i, u_irangle\
&= sum_{i=1}^m leftlangle sum_{j=1}^mlangle u_i,v_jrangle A^*A v_j , sum_{k=1}^mlangle u_i,v_krangle v_krightrangle\
&= sum_{j=1}^m sum_{k=1}^m left(sum_{i=1}^mlangle u_i,v_jrangle langle v_k,u_irangleright)langle A^*A v_j,v_krangle\
&= sum_{j=1}^m sum_{k=1}^m langle v_j,v_kranglelangle A^*A v_j,v_krangle\
&= sum_{j=1}^m langle A^*A v_j,v_jrangle\
&= sum_{j=1}^m |Av_j|_2^2
end{align}
Hence we can extend any orthonormal set ${v_1, ldots, v_k} subseteq mathbb{R}^m$ to an orthonormal basis ${v_1, ldots, v_m}$ for $mathbb{R}^m$ to obtain
$$sum_{i=1}^k |Av_i|_2^2 lesum_{i=1}^m |Av_i|_2^2 = sum_{i=1}^m |Au_i|_2^2 = |A|_F^2 $$
add a comment |
Note that $A^*A$ is a square matrix and $A^*A ge 0$ so there exists an orthonormal basis ${u_1, ldots, u_m}$ for $mathbb{R}^m$ such that $A^*A u_i = lambda_i u_i$ for some $lambda ge 0$.
We have
$$sum_{i=1}^m |Au_i|_2^2 = sum_{i=1}^m langle Au_i, Au_irangle = sum_{i=1}^m langle A^*Au_i, u_irangle = sum_{i=1}^m lambda_i =operatorname{Tr}(A^*A) = |A|_F^2$$
The interesting part is that the sum $sum_{i=1}^m |Au_i|_2^2$ is actually independent of the choice of the orthonormal basis ${u_1, ldots, u_m}$. Indeed, if ${v_1, ldots, v_m}$ is some other orthonormal basis for $mathbb{R}^m$, we have
begin{align}
sum_{i=1}^m |Au_i|_2^2 &= sum_{i=1}^m langle A^*Au_i, u_irangle\
&= sum_{i=1}^m leftlangle sum_{j=1}^mlangle u_i,v_jrangle A^*A v_j , sum_{k=1}^mlangle u_i,v_krangle v_krightrangle\
&= sum_{j=1}^m sum_{k=1}^m left(sum_{i=1}^mlangle u_i,v_jrangle langle v_k,u_irangleright)langle A^*A v_j,v_krangle\
&= sum_{j=1}^m sum_{k=1}^m langle v_j,v_kranglelangle A^*A v_j,v_krangle\
&= sum_{j=1}^m langle A^*A v_j,v_jrangle\
&= sum_{j=1}^m |Av_j|_2^2
end{align}
Hence we can extend any orthonormal set ${v_1, ldots, v_k} subseteq mathbb{R}^m$ to an orthonormal basis ${v_1, ldots, v_m}$ for $mathbb{R}^m$ to obtain
$$sum_{i=1}^k |Av_i|_2^2 lesum_{i=1}^m |Av_i|_2^2 = sum_{i=1}^m |Au_i|_2^2 = |A|_F^2 $$
add a comment |
Note that $A^*A$ is a square matrix and $A^*A ge 0$ so there exists an orthonormal basis ${u_1, ldots, u_m}$ for $mathbb{R}^m$ such that $A^*A u_i = lambda_i u_i$ for some $lambda ge 0$.
We have
$$sum_{i=1}^m |Au_i|_2^2 = sum_{i=1}^m langle Au_i, Au_irangle = sum_{i=1}^m langle A^*Au_i, u_irangle = sum_{i=1}^m lambda_i =operatorname{Tr}(A^*A) = |A|_F^2$$
The interesting part is that the sum $sum_{i=1}^m |Au_i|_2^2$ is actually independent of the choice of the orthonormal basis ${u_1, ldots, u_m}$. Indeed, if ${v_1, ldots, v_m}$ is some other orthonormal basis for $mathbb{R}^m$, we have
begin{align}
sum_{i=1}^m |Au_i|_2^2 &= sum_{i=1}^m langle A^*Au_i, u_irangle\
&= sum_{i=1}^m leftlangle sum_{j=1}^mlangle u_i,v_jrangle A^*A v_j , sum_{k=1}^mlangle u_i,v_krangle v_krightrangle\
&= sum_{j=1}^m sum_{k=1}^m left(sum_{i=1}^mlangle u_i,v_jrangle langle v_k,u_irangleright)langle A^*A v_j,v_krangle\
&= sum_{j=1}^m sum_{k=1}^m langle v_j,v_kranglelangle A^*A v_j,v_krangle\
&= sum_{j=1}^m langle A^*A v_j,v_jrangle\
&= sum_{j=1}^m |Av_j|_2^2
end{align}
Hence we can extend any orthonormal set ${v_1, ldots, v_k} subseteq mathbb{R}^m$ to an orthonormal basis ${v_1, ldots, v_m}$ for $mathbb{R}^m$ to obtain
$$sum_{i=1}^k |Av_i|_2^2 lesum_{i=1}^m |Av_i|_2^2 = sum_{i=1}^m |Au_i|_2^2 = |A|_F^2 $$
Note that $A^*A$ is a square matrix and $A^*A ge 0$ so there exists an orthonormal basis ${u_1, ldots, u_m}$ for $mathbb{R}^m$ such that $A^*A u_i = lambda_i u_i$ for some $lambda ge 0$.
We have
$$sum_{i=1}^m |Au_i|_2^2 = sum_{i=1}^m langle Au_i, Au_irangle = sum_{i=1}^m langle A^*Au_i, u_irangle = sum_{i=1}^m lambda_i =operatorname{Tr}(A^*A) = |A|_F^2$$
The interesting part is that the sum $sum_{i=1}^m |Au_i|_2^2$ is actually independent of the choice of the orthonormal basis ${u_1, ldots, u_m}$. Indeed, if ${v_1, ldots, v_m}$ is some other orthonormal basis for $mathbb{R}^m$, we have
begin{align}
sum_{i=1}^m |Au_i|_2^2 &= sum_{i=1}^m langle A^*Au_i, u_irangle\
&= sum_{i=1}^m leftlangle sum_{j=1}^mlangle u_i,v_jrangle A^*A v_j , sum_{k=1}^mlangle u_i,v_krangle v_krightrangle\
&= sum_{j=1}^m sum_{k=1}^m left(sum_{i=1}^mlangle u_i,v_jrangle langle v_k,u_irangleright)langle A^*A v_j,v_krangle\
&= sum_{j=1}^m sum_{k=1}^m langle v_j,v_kranglelangle A^*A v_j,v_krangle\
&= sum_{j=1}^m langle A^*A v_j,v_jrangle\
&= sum_{j=1}^m |Av_j|_2^2
end{align}
Hence we can extend any orthonormal set ${v_1, ldots, v_k} subseteq mathbb{R}^m$ to an orthonormal basis ${v_1, ldots, v_m}$ for $mathbb{R}^m$ to obtain
$$sum_{i=1}^k |Av_i|_2^2 lesum_{i=1}^m |Av_i|_2^2 = sum_{i=1}^m |Au_i|_2^2 = |A|_F^2 $$
answered yesterday
mechanodroid
26.9k62447
26.9k62447
add a comment |
add a comment |
Thanks for contributing an answer to Mathematics Stack Exchange!
- Please be sure to answer the question. Provide details and share your research!
But avoid …
- Asking for help, clarification, or responding to other answers.
- Making statements based on opinion; back them up with references or personal experience.
Use MathJax to format equations. MathJax reference.
To learn more, see our tips on writing great answers.
Some of your past answers have not been well-received, and you're in danger of being blocked from answering.
Please pay close attention to the following guidance:
- Please be sure to answer the question. Provide details and share your research!
But avoid …
- Asking for help, clarification, or responding to other answers.
- Making statements based on opinion; back them up with references or personal experience.
To learn more, see our tips on writing great answers.
Sign up or log in
StackExchange.ready(function () {
StackExchange.helpers.onClickDraftSave('#login-link');
});
Sign up using Google
Sign up using Facebook
Sign up using Email and Password
Post as a guest
Required, but never shown
StackExchange.ready(
function () {
StackExchange.openid.initPostLogin('.new-post-login', 'https%3a%2f%2fmath.stackexchange.com%2fquestions%2f3060689%2fhow-to-show-for-any-orthogonal-vector-set-and-any-matrix-sum-u-au-22-leq%23new-answer', 'question_page');
}
);
Post as a guest
Required, but never shown
Sign up or log in
StackExchange.ready(function () {
StackExchange.helpers.onClickDraftSave('#login-link');
});
Sign up using Google
Sign up using Facebook
Sign up using Email and Password
Post as a guest
Required, but never shown
Sign up or log in
StackExchange.ready(function () {
StackExchange.helpers.onClickDraftSave('#login-link');
});
Sign up using Google
Sign up using Facebook
Sign up using Email and Password
Post as a guest
Required, but never shown
Sign up or log in
StackExchange.ready(function () {
StackExchange.helpers.onClickDraftSave('#login-link');
});
Sign up using Google
Sign up using Facebook
Sign up using Email and Password
Sign up using Google
Sign up using Facebook
Sign up using Email and Password
Post as a guest
Required, but never shown
Required, but never shown
Required, but never shown
Required, but never shown
Required, but never shown
Required, but never shown
Required, but never shown
Required, but never shown
Required, but never shown
53rKyzU JEe7 swlyMZ7nux pWPMe,5W9IE MdWrdaYwJu8 LhWtd,IZHofxMzWhNiU5M5XZL,1w9Pkm,qCToE C
1
$|AU|_F=|A|_F$ which follows from the trace property and $UU^*=I$.
– A.Γ.
2 days ago
1
But they are not orthanormal, it says they are orthogonal.
– Saeed
2 days ago
Some authors are sloppy about the distinction between "orthonormal" and "orthogonal". It's pretty straight-forward to normalize orthogonal vectors (orthogonalizing them using, say, Gram-Schmidt, is what takes all the effort!), so some authors don't trouble about it. Technically, you're correct, of course.
– Adrian Keister
2 days ago
2
It is sloppy written, but $U$ matrix they work with in this proof has orthonormal columns (Observation 14, page 14). Otherwise it is not true (take one vector and multiply it by a huge number).
– A.Γ.
2 days ago