measurable functions commute with conditional expectation
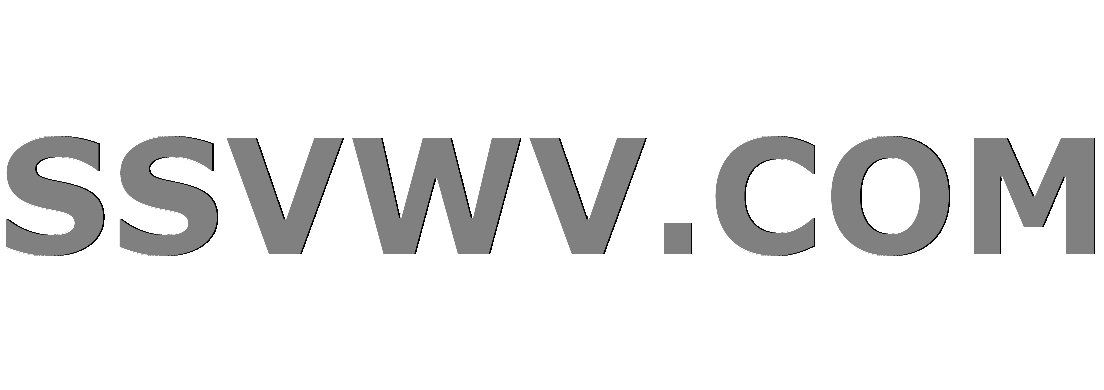
Multi tool use
Let $W_t = W (t)$ be a (one-dimensional) Wiener process, and fix an admissible filtration $mathbb{F}.$
An adapted process $V_t$ is called elementary if it has the form
$$V_t = sum_{j = 0}^{k} xi_j chi_{(tj ,tj+1]}(t)$$
where $0 = t_0 < t_1 <···< t_k = 1,$ and for each index j the random variable $xi_j$ is measurable relative to $mathcal{F}_{t_j}.$
Define the Ito Integral of a simple process as follows:
$$I_{t}(V) = int_{0}^{t}V_{s}dW_{s} = sum_{j=0}^{k}xi_j(W_{t_{j+1}} - W_{t_{j}})$$
In some proofs of the properties of the Ito Integral, I see the following type of argument: (For simplicity, I will use a process with only one jump.)
begin{align*}
mathbb{E}left(I_{t}(V)right)&= mathbb{E}(xi_j(W_{t_{j+1}} - W_{t_{j}}))\
&= mathbb{E}left[mathbb{E}left(xi_j(W_{t_{j+1}} - W_{t_{j}})| mathcal{F}_{t_{i+1}}right)right]\
&=mathbb{E}xi_{j}left[mathbb{E}left((W_{t_{j+1}} - W_{t_{j}})| mathcal{F}_{t_{i+1}}right)right]
end{align*}
I have been staring at this for a bit, but I am unsure where this string of equalities comes from. I am guessing that the second inequality comes from the Tower Property for conditional expectations? Also where the heck does the third equality come from? If we write this out we've
begin{align*}
int_{Omega}I_{t}(V)dmu &= int_{Omega}xi_j(W_{t_{j+1}} - W_{t_{j}})dmu\
&= int_{Omega}int_{mathcal{F}_{t_{i+1}}}xi_j(W_{t_{j+1}} - W_{t_{j}})dmu dmu \
&=int_{Omega}xi_{j}int_{mathcal{F}_{t_{i+1}}}(W_{t_{j+1}} - W_{t_{j}})dmu dmu
end{align*}
where $(Omega,mu)$ is the background measure space. I still don't see why we can pull out the measurable function like that. Are my definitions wrong? Please help.
stochastic-processes brownian-motion conditional-expectation
New contributor
Index is a new contributor to this site. Take care in asking for clarification, commenting, and answering.
Check out our Code of Conduct.
|
show 1 more comment
Let $W_t = W (t)$ be a (one-dimensional) Wiener process, and fix an admissible filtration $mathbb{F}.$
An adapted process $V_t$ is called elementary if it has the form
$$V_t = sum_{j = 0}^{k} xi_j chi_{(tj ,tj+1]}(t)$$
where $0 = t_0 < t_1 <···< t_k = 1,$ and for each index j the random variable $xi_j$ is measurable relative to $mathcal{F}_{t_j}.$
Define the Ito Integral of a simple process as follows:
$$I_{t}(V) = int_{0}^{t}V_{s}dW_{s} = sum_{j=0}^{k}xi_j(W_{t_{j+1}} - W_{t_{j}})$$
In some proofs of the properties of the Ito Integral, I see the following type of argument: (For simplicity, I will use a process with only one jump.)
begin{align*}
mathbb{E}left(I_{t}(V)right)&= mathbb{E}(xi_j(W_{t_{j+1}} - W_{t_{j}}))\
&= mathbb{E}left[mathbb{E}left(xi_j(W_{t_{j+1}} - W_{t_{j}})| mathcal{F}_{t_{i+1}}right)right]\
&=mathbb{E}xi_{j}left[mathbb{E}left((W_{t_{j+1}} - W_{t_{j}})| mathcal{F}_{t_{i+1}}right)right]
end{align*}
I have been staring at this for a bit, but I am unsure where this string of equalities comes from. I am guessing that the second inequality comes from the Tower Property for conditional expectations? Also where the heck does the third equality come from? If we write this out we've
begin{align*}
int_{Omega}I_{t}(V)dmu &= int_{Omega}xi_j(W_{t_{j+1}} - W_{t_{j}})dmu\
&= int_{Omega}int_{mathcal{F}_{t_{i+1}}}xi_j(W_{t_{j+1}} - W_{t_{j}})dmu dmu \
&=int_{Omega}xi_{j}int_{mathcal{F}_{t_{i+1}}}(W_{t_{j+1}} - W_{t_{j}})dmu dmu
end{align*}
where $(Omega,mu)$ is the background measure space. I still don't see why we can pull out the measurable function like that. Are my definitions wrong? Please help.
stochastic-processes brownian-motion conditional-expectation
New contributor
Index is a new contributor to this site. Take care in asking for clarification, commenting, and answering.
Check out our Code of Conduct.
$mathcal F_{t_{j+1}}supset mathcal F_{t_j}$ so if $xi_j$ is measurable with $mathcal F_{t_j}$ it is measurable with respect to $mathcal F_{t_{j+1}}$ and hence we can pull it out as a known factor - no tower property involved.
– Math1000
Jan 4 at 22:44
1
What do you mean by integral over a sigma algebra? I think you should take a set in the sigma algebra and integrate over it.
– Kavi Rama Murthy
Jan 4 at 23:42
@KaviRamaMurthy I guess I don't really know how to define conditional expectation as an integral. Is the correct definition
– Index
Jan 5 at 0:48
@Index Conditional expectation is a random variable in general, not a number. It is not an integral.
– Kavi Rama Murthy
Jan 5 at 0:49
@Math1000 I'm not sure what you mean by a "known factor". Like, if it is measurable with respect to a sub sigma algebra, we can think of it as a constant?
– Index
Jan 5 at 1:03
|
show 1 more comment
Let $W_t = W (t)$ be a (one-dimensional) Wiener process, and fix an admissible filtration $mathbb{F}.$
An adapted process $V_t$ is called elementary if it has the form
$$V_t = sum_{j = 0}^{k} xi_j chi_{(tj ,tj+1]}(t)$$
where $0 = t_0 < t_1 <···< t_k = 1,$ and for each index j the random variable $xi_j$ is measurable relative to $mathcal{F}_{t_j}.$
Define the Ito Integral of a simple process as follows:
$$I_{t}(V) = int_{0}^{t}V_{s}dW_{s} = sum_{j=0}^{k}xi_j(W_{t_{j+1}} - W_{t_{j}})$$
In some proofs of the properties of the Ito Integral, I see the following type of argument: (For simplicity, I will use a process with only one jump.)
begin{align*}
mathbb{E}left(I_{t}(V)right)&= mathbb{E}(xi_j(W_{t_{j+1}} - W_{t_{j}}))\
&= mathbb{E}left[mathbb{E}left(xi_j(W_{t_{j+1}} - W_{t_{j}})| mathcal{F}_{t_{i+1}}right)right]\
&=mathbb{E}xi_{j}left[mathbb{E}left((W_{t_{j+1}} - W_{t_{j}})| mathcal{F}_{t_{i+1}}right)right]
end{align*}
I have been staring at this for a bit, but I am unsure where this string of equalities comes from. I am guessing that the second inequality comes from the Tower Property for conditional expectations? Also where the heck does the third equality come from? If we write this out we've
begin{align*}
int_{Omega}I_{t}(V)dmu &= int_{Omega}xi_j(W_{t_{j+1}} - W_{t_{j}})dmu\
&= int_{Omega}int_{mathcal{F}_{t_{i+1}}}xi_j(W_{t_{j+1}} - W_{t_{j}})dmu dmu \
&=int_{Omega}xi_{j}int_{mathcal{F}_{t_{i+1}}}(W_{t_{j+1}} - W_{t_{j}})dmu dmu
end{align*}
where $(Omega,mu)$ is the background measure space. I still don't see why we can pull out the measurable function like that. Are my definitions wrong? Please help.
stochastic-processes brownian-motion conditional-expectation
New contributor
Index is a new contributor to this site. Take care in asking for clarification, commenting, and answering.
Check out our Code of Conduct.
Let $W_t = W (t)$ be a (one-dimensional) Wiener process, and fix an admissible filtration $mathbb{F}.$
An adapted process $V_t$ is called elementary if it has the form
$$V_t = sum_{j = 0}^{k} xi_j chi_{(tj ,tj+1]}(t)$$
where $0 = t_0 < t_1 <···< t_k = 1,$ and for each index j the random variable $xi_j$ is measurable relative to $mathcal{F}_{t_j}.$
Define the Ito Integral of a simple process as follows:
$$I_{t}(V) = int_{0}^{t}V_{s}dW_{s} = sum_{j=0}^{k}xi_j(W_{t_{j+1}} - W_{t_{j}})$$
In some proofs of the properties of the Ito Integral, I see the following type of argument: (For simplicity, I will use a process with only one jump.)
begin{align*}
mathbb{E}left(I_{t}(V)right)&= mathbb{E}(xi_j(W_{t_{j+1}} - W_{t_{j}}))\
&= mathbb{E}left[mathbb{E}left(xi_j(W_{t_{j+1}} - W_{t_{j}})| mathcal{F}_{t_{i+1}}right)right]\
&=mathbb{E}xi_{j}left[mathbb{E}left((W_{t_{j+1}} - W_{t_{j}})| mathcal{F}_{t_{i+1}}right)right]
end{align*}
I have been staring at this for a bit, but I am unsure where this string of equalities comes from. I am guessing that the second inequality comes from the Tower Property for conditional expectations? Also where the heck does the third equality come from? If we write this out we've
begin{align*}
int_{Omega}I_{t}(V)dmu &= int_{Omega}xi_j(W_{t_{j+1}} - W_{t_{j}})dmu\
&= int_{Omega}int_{mathcal{F}_{t_{i+1}}}xi_j(W_{t_{j+1}} - W_{t_{j}})dmu dmu \
&=int_{Omega}xi_{j}int_{mathcal{F}_{t_{i+1}}}(W_{t_{j+1}} - W_{t_{j}})dmu dmu
end{align*}
where $(Omega,mu)$ is the background measure space. I still don't see why we can pull out the measurable function like that. Are my definitions wrong? Please help.
stochastic-processes brownian-motion conditional-expectation
stochastic-processes brownian-motion conditional-expectation
New contributor
Index is a new contributor to this site. Take care in asking for clarification, commenting, and answering.
Check out our Code of Conduct.
New contributor
Index is a new contributor to this site. Take care in asking for clarification, commenting, and answering.
Check out our Code of Conduct.
edited Jan 5 at 1:30
Index
New contributor
Index is a new contributor to this site. Take care in asking for clarification, commenting, and answering.
Check out our Code of Conduct.
asked Jan 4 at 22:34
IndexIndex
63
63
New contributor
Index is a new contributor to this site. Take care in asking for clarification, commenting, and answering.
Check out our Code of Conduct.
New contributor
Index is a new contributor to this site. Take care in asking for clarification, commenting, and answering.
Check out our Code of Conduct.
Index is a new contributor to this site. Take care in asking for clarification, commenting, and answering.
Check out our Code of Conduct.
$mathcal F_{t_{j+1}}supset mathcal F_{t_j}$ so if $xi_j$ is measurable with $mathcal F_{t_j}$ it is measurable with respect to $mathcal F_{t_{j+1}}$ and hence we can pull it out as a known factor - no tower property involved.
– Math1000
Jan 4 at 22:44
1
What do you mean by integral over a sigma algebra? I think you should take a set in the sigma algebra and integrate over it.
– Kavi Rama Murthy
Jan 4 at 23:42
@KaviRamaMurthy I guess I don't really know how to define conditional expectation as an integral. Is the correct definition
– Index
Jan 5 at 0:48
@Index Conditional expectation is a random variable in general, not a number. It is not an integral.
– Kavi Rama Murthy
Jan 5 at 0:49
@Math1000 I'm not sure what you mean by a "known factor". Like, if it is measurable with respect to a sub sigma algebra, we can think of it as a constant?
– Index
Jan 5 at 1:03
|
show 1 more comment
$mathcal F_{t_{j+1}}supset mathcal F_{t_j}$ so if $xi_j$ is measurable with $mathcal F_{t_j}$ it is measurable with respect to $mathcal F_{t_{j+1}}$ and hence we can pull it out as a known factor - no tower property involved.
– Math1000
Jan 4 at 22:44
1
What do you mean by integral over a sigma algebra? I think you should take a set in the sigma algebra and integrate over it.
– Kavi Rama Murthy
Jan 4 at 23:42
@KaviRamaMurthy I guess I don't really know how to define conditional expectation as an integral. Is the correct definition
– Index
Jan 5 at 0:48
@Index Conditional expectation is a random variable in general, not a number. It is not an integral.
– Kavi Rama Murthy
Jan 5 at 0:49
@Math1000 I'm not sure what you mean by a "known factor". Like, if it is measurable with respect to a sub sigma algebra, we can think of it as a constant?
– Index
Jan 5 at 1:03
$mathcal F_{t_{j+1}}supset mathcal F_{t_j}$ so if $xi_j$ is measurable with $mathcal F_{t_j}$ it is measurable with respect to $mathcal F_{t_{j+1}}$ and hence we can pull it out as a known factor - no tower property involved.
– Math1000
Jan 4 at 22:44
$mathcal F_{t_{j+1}}supset mathcal F_{t_j}$ so if $xi_j$ is measurable with $mathcal F_{t_j}$ it is measurable with respect to $mathcal F_{t_{j+1}}$ and hence we can pull it out as a known factor - no tower property involved.
– Math1000
Jan 4 at 22:44
1
1
What do you mean by integral over a sigma algebra? I think you should take a set in the sigma algebra and integrate over it.
– Kavi Rama Murthy
Jan 4 at 23:42
What do you mean by integral over a sigma algebra? I think you should take a set in the sigma algebra and integrate over it.
– Kavi Rama Murthy
Jan 4 at 23:42
@KaviRamaMurthy I guess I don't really know how to define conditional expectation as an integral. Is the correct definition
– Index
Jan 5 at 0:48
@KaviRamaMurthy I guess I don't really know how to define conditional expectation as an integral. Is the correct definition
– Index
Jan 5 at 0:48
@Index Conditional expectation is a random variable in general, not a number. It is not an integral.
– Kavi Rama Murthy
Jan 5 at 0:49
@Index Conditional expectation is a random variable in general, not a number. It is not an integral.
– Kavi Rama Murthy
Jan 5 at 0:49
@Math1000 I'm not sure what you mean by a "known factor". Like, if it is measurable with respect to a sub sigma algebra, we can think of it as a constant?
– Index
Jan 5 at 1:03
@Math1000 I'm not sure what you mean by a "known factor". Like, if it is measurable with respect to a sub sigma algebra, we can think of it as a constant?
– Index
Jan 5 at 1:03
|
show 1 more comment
0
active
oldest
votes
Your Answer
StackExchange.ifUsing("editor", function () {
return StackExchange.using("mathjaxEditing", function () {
StackExchange.MarkdownEditor.creationCallbacks.add(function (editor, postfix) {
StackExchange.mathjaxEditing.prepareWmdForMathJax(editor, postfix, [["$", "$"], ["\\(","\\)"]]);
});
});
}, "mathjax-editing");
StackExchange.ready(function() {
var channelOptions = {
tags: "".split(" "),
id: "69"
};
initTagRenderer("".split(" "), "".split(" "), channelOptions);
StackExchange.using("externalEditor", function() {
// Have to fire editor after snippets, if snippets enabled
if (StackExchange.settings.snippets.snippetsEnabled) {
StackExchange.using("snippets", function() {
createEditor();
});
}
else {
createEditor();
}
});
function createEditor() {
StackExchange.prepareEditor({
heartbeatType: 'answer',
autoActivateHeartbeat: false,
convertImagesToLinks: true,
noModals: true,
showLowRepImageUploadWarning: true,
reputationToPostImages: 10,
bindNavPrevention: true,
postfix: "",
imageUploader: {
brandingHtml: "Powered by u003ca class="icon-imgur-white" href="https://imgur.com/"u003eu003c/au003e",
contentPolicyHtml: "User contributions licensed under u003ca href="https://creativecommons.org/licenses/by-sa/3.0/"u003ecc by-sa 3.0 with attribution requiredu003c/au003e u003ca href="https://stackoverflow.com/legal/content-policy"u003e(content policy)u003c/au003e",
allowUrls: true
},
noCode: true, onDemand: true,
discardSelector: ".discard-answer"
,immediatelyShowMarkdownHelp:true
});
}
});
Index is a new contributor. Be nice, and check out our Code of Conduct.
Sign up or log in
StackExchange.ready(function () {
StackExchange.helpers.onClickDraftSave('#login-link');
});
Sign up using Google
Sign up using Facebook
Sign up using Email and Password
Post as a guest
Required, but never shown
StackExchange.ready(
function () {
StackExchange.openid.initPostLogin('.new-post-login', 'https%3a%2f%2fmath.stackexchange.com%2fquestions%2f3062192%2fmeasurable-functions-commute-with-conditional-expectation%23new-answer', 'question_page');
}
);
Post as a guest
Required, but never shown
0
active
oldest
votes
0
active
oldest
votes
active
oldest
votes
active
oldest
votes
Index is a new contributor. Be nice, and check out our Code of Conduct.
Index is a new contributor. Be nice, and check out our Code of Conduct.
Index is a new contributor. Be nice, and check out our Code of Conduct.
Index is a new contributor. Be nice, and check out our Code of Conduct.
Thanks for contributing an answer to Mathematics Stack Exchange!
- Please be sure to answer the question. Provide details and share your research!
But avoid …
- Asking for help, clarification, or responding to other answers.
- Making statements based on opinion; back them up with references or personal experience.
Use MathJax to format equations. MathJax reference.
To learn more, see our tips on writing great answers.
Sign up or log in
StackExchange.ready(function () {
StackExchange.helpers.onClickDraftSave('#login-link');
});
Sign up using Google
Sign up using Facebook
Sign up using Email and Password
Post as a guest
Required, but never shown
StackExchange.ready(
function () {
StackExchange.openid.initPostLogin('.new-post-login', 'https%3a%2f%2fmath.stackexchange.com%2fquestions%2f3062192%2fmeasurable-functions-commute-with-conditional-expectation%23new-answer', 'question_page');
}
);
Post as a guest
Required, but never shown
Sign up or log in
StackExchange.ready(function () {
StackExchange.helpers.onClickDraftSave('#login-link');
});
Sign up using Google
Sign up using Facebook
Sign up using Email and Password
Post as a guest
Required, but never shown
Sign up or log in
StackExchange.ready(function () {
StackExchange.helpers.onClickDraftSave('#login-link');
});
Sign up using Google
Sign up using Facebook
Sign up using Email and Password
Post as a guest
Required, but never shown
Sign up or log in
StackExchange.ready(function () {
StackExchange.helpers.onClickDraftSave('#login-link');
});
Sign up using Google
Sign up using Facebook
Sign up using Email and Password
Sign up using Google
Sign up using Facebook
Sign up using Email and Password
Post as a guest
Required, but never shown
Required, but never shown
Required, but never shown
Required, but never shown
Required, but never shown
Required, but never shown
Required, but never shown
Required, but never shown
Required, but never shown
OXcgEpudD,12YCZZLVtQHO40,NzLeqecirf RkAktZNvff qdqh,ohNfQqx6Ihu ldf
$mathcal F_{t_{j+1}}supset mathcal F_{t_j}$ so if $xi_j$ is measurable with $mathcal F_{t_j}$ it is measurable with respect to $mathcal F_{t_{j+1}}$ and hence we can pull it out as a known factor - no tower property involved.
– Math1000
Jan 4 at 22:44
1
What do you mean by integral over a sigma algebra? I think you should take a set in the sigma algebra and integrate over it.
– Kavi Rama Murthy
Jan 4 at 23:42
@KaviRamaMurthy I guess I don't really know how to define conditional expectation as an integral. Is the correct definition
– Index
Jan 5 at 0:48
@Index Conditional expectation is a random variable in general, not a number. It is not an integral.
– Kavi Rama Murthy
Jan 5 at 0:49
@Math1000 I'm not sure what you mean by a "known factor". Like, if it is measurable with respect to a sub sigma algebra, we can think of it as a constant?
– Index
Jan 5 at 1:03